What is conjoint analysis? The complete guide
Conjoint analysis is an advanced market research method that gets under the skin of how people make decisions. It is used to quantify what customers really value in products and services to create models and forecasts based on presenting people with realistic product choices and then analysing what features most drive purchasing decisions.
Conjoint analysis is used to build market models and forecasts to answer questions such as "Should we build in more features, or change our prices?" or "Which of these changes will hurt our competitors most?", or "What is the optimum price to charge?" that allow the business to optimise product and service design to customer needs.
To explore or play with conjoint analysis, try our interactive Conjoint Demonstration, our simple conjoint in Excel to see how conjoint analysis works numerically, or use our free, full-featured Conjoint Explorer to design and test your own conjoint experiments.
Conjoint overview
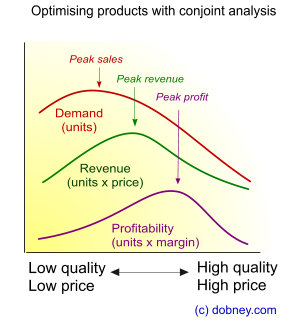
Conjoint analysis aims to find the optimum positioning between low-price-low-quality and high-price-high-quality in terms of price and features by quantifying the trade-offs and compromises customers take in decision making.
Every customer making choices between products and services is faced with trade-offs (see our conjoint demonstration). Is high quality more important than a low price and quick delivery for instance? Or is good service more important than design and looks?
For businesses, understanding precisely how customers value different elements of a product or service allows product development to be optimised to give the best balance of features or quality, for the prices the customer is willing to pay. At a market level, conjoint analysis can be used to identify the best product range for different segments or market needs, by determining which features, value and price, across a set of products, would maximise customer value and market returns.
Conjoint analysis is also known as Discrete Choice Estimation, or stated preference research and is one of a range of trade-off based research techniques.
An established and powerful means of estimating customer value
With on-going development and improvements since it was invented in the 1970s conjoint analysis has become a core tool for product planning and pricing research. By understanding precisely how people make decisions and what they value in your products and services, you can work out the sweetspot or optimum level of features and services that balance value to the customer against cost to the company and forecast potential demand or market share in a competitive market situation.
It is, however, a sophisticated technique and expertise is required to ensure the design and outputs will achieve the business objectives.
Conjoint principles - attributes and levels
The principles behind conjoint analysis start with breaking a product or service down into it's constituent parts (called attributes and levels - see the section on how to design a conjoint analysis study) then to test combinations of these parts in order to find out what customers prefer. By designing the study appropriately using carefully chosen statistical designs (also known as experimental designs) it is then possible to use statistical analysis to work out the value, or utility score, of each part of the product or service in terms of its contribution to the customer's decision.
For example a computer may be described in terms of attributes such as processor type, hard disk size and amount of memory. Each of these attributes is broken down into levels - for instance levels of the attribute for memory size might be 1GB, 2GB, 3GB and 4GB. Play with attribute and levels in our Conjoint Explorer to see how designs can be created.
From attributes and levels to product profiles and choice tasks
These attributes and levels can be used to define different products by choosing different levels for different products so the first stage in conjoint analysis is to create a set of product profiles (possible combinations of attributes and levels) to produce a set of options from which customers or respondents are then asked to choose - know as choice sets or choice tasks.
Obviously, the number of potential profiles increases rapidly for every new attribute added as the number of possible combinations increases, so there are statistical techniques and design methods to simplify both the number of profiles to be tested and the way in which preferences are tested so that the maximum amount of choice information can be collected from the smallest set of choice tasks.
Choosing the right type or flavour of conjoint analysis
The precise approach to creating 'choice tasks' depends on the which type or flavours of conjoint analysis is most appropriate to use. The most common approach is choice-based conjoint (CBC), but other flavours exist. Students often get taught full-profile conjoint using ratings or cards, for more attributes adaptive designs get used, such as adaptive conjoint analysis (ACA), menu-based conjoint, or adaptive choice based conjoint (ACBC). Economists might look at Stated Preference or Discrete Choice Methods.
Conjoint analysis might not be the right option. Other approaches such as MaxDiff, Simalto or hierarchy of needs studies, each have different ways to manage the balance between the number of attributes that can be included and the relative complexity of the choices that need to be shown in order to get good quality data.
Statistical design and analysis
A conjoint analysis study relies on appropriate statistical design in order to be able to estimate the utility models. Once all the choice tasks have been completed, analysis involves modelling what drove customers choices or preferences from the product profiles offered.
The statistical output then quantifies both what is driving the preference from the attributes and levels shown - known as utilities or part-worths and importance scores. These utilities give an measurement of value for each level, of each attribute, in terms of its contribution to the choices that were made and so shows the relative value of one level against another.
Market models - forecasting market potential
The statistical output gives a detailed quantified picture of how customers make decisions, and a set of data that can be used to build market models which can predict preferences or estimate market share in new market conditions in order to forecast the impact of product or service changes on the market. For businesses this allows them to see where and how they can gain the greatest improvements over their competitors, where they can add value for the customer, how price impacts on decisions and so forecast demand and revenue. Not surprisingly conjoint analysis has become a key tool in building and developing market strategies.
By combining these market models with internal project costings, companies can evaluate decisions in terms of Return on Investment (ROI) before going to market. For example determining what resources to put into New Product Development and in what areas. Choice-based conjoint or discrete choice modelling also form the basis of much pricing research and powerful needs-based segmentation.
"We were looking for an agency that could understand our solutions and complex customer base in order to transfer this understanding into a comprehensive customer survey.
dobney.com quickly gained deep insight into the specificities of our business and designed an excellent, state-of-the-art conjoint survey. They delivered professional and individual service of a quality we had never experienced before. It was great working with dobney.com and the findings derived from the survey are invaluable for us."
Marketing Manager, Leica Microsystems
Alternatives to conjoint - from MaxDiff to configurators and e-commerce mock-ups
Conjoint analysis is relatively complex as it requires an understanding of how to use and create attributes and levels, what flavour to use, how to make the product profiles, what choice task to offer and then how to analyse the data and build the market model. It is possible to use off-the-shelf software which will provide guidance and help, but it can be also make it easy to make mistakes or generate poor designs. And conjoint analysis doesn't always fit, particularly if there are many levels, or a deeper more emotional drive to decision making. So, depending on the product or service, it is possible that off-the-shelf approaches aren't always suitable and other methods are needed. Fortunately there are a number of related approaches used as alternatives to conjoint analysis, such as MaxDiff, configurators or Simalto (also known as trade-off grids). MaxDiff is more about measuring the value from a list of items, than generating complete products, but it uses many of the same features and analytics as conjoint. Simalto, like conjoint analysis, breaks products down into attributes and levels, but then presents them as a grid of options to respondents.
A range of other research techniques including menu building (building a configured product from a range of selected options), and search and filter studies in the form of e-commerce style mock-ups where respondents hunt for their most preferred products can also be used in conjunction with or as alternatives to conjoint analysis.
Demonstrations and further reading
To see the mathematical workings, we have a fully worked up simple conjoint analysis worked example in Excel to show how conjoint analysis functions mathematically to estimate part-worths or utilities from design to analysis. Or just play with our Pizza demonstration which shows how utility estimations arise from choices about pizza preferences.
- See our interactive instant conjoint analysis demonstration showing how customer value can be calculated from choices.
- See how conjoint market models and simulators work to enable better ROI decisions based on customer values.
- Explore conjoint analysis in-depth with our online Conjoint Explorer which allows you to design and test your own attributes and levels and choice tasks, and see results.
- Conjoint analysis design principles
- Conjoint analysis types or flavours
For help and advice on using conjoint analysis for market modelling, or to carry out conjoint analysis research email info@dobney.com